Generative AI, a subset of artificial intelligence, uses machine learning models to generate new content, including text, images, videos, and audio, based on patterns and data learned from existing examples. Examples include chatbots like ChatGPT, image generators like DALL-E, and video generators like Runway’s Gen-2.
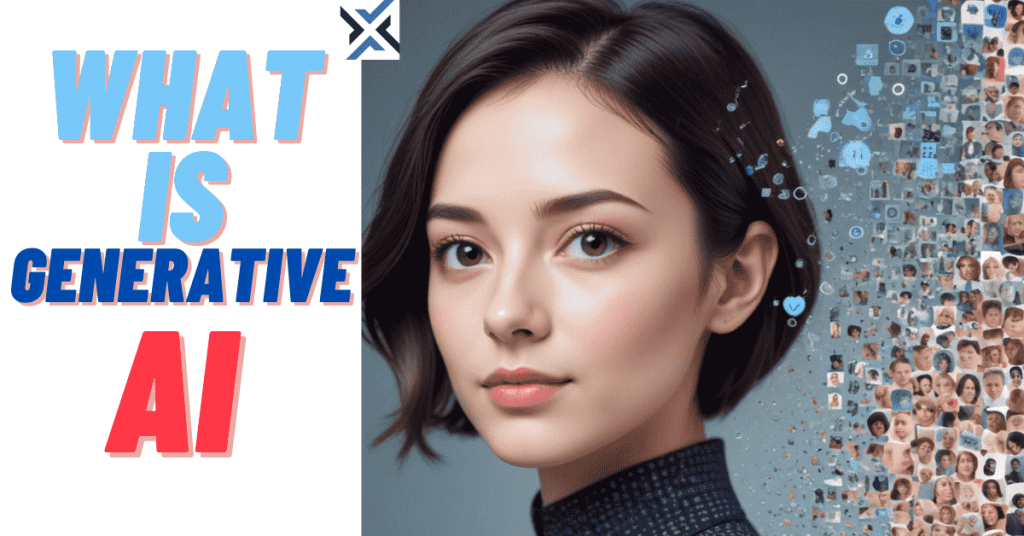
Corrections and Clarifications:
- Artificial Intelligence vs. Generative AI:
- Artificial Intelligence (AI): A broad field encompassing various techniques and models designed to simulate human intelligence, including decision-making, problem-solving, and learning.
- Generative AI: A specific subset of AI focused on generating new content based on learned data patterns.
- Machine Learning Models:
- Generative AI often uses specific types of machine learning models, such as GANs, VAEs, and transformers, to create new content.
- Examples of Generative AI:
- ChatGPT: A generative AI model for text, developed by OpenAI.
- DALL-E: An image generator that creates new images from textual descriptions.
- Runway’s Gen-2: An example of a video generator using generative AI techniques. (Note: “Sora12” is not a widely recognized example in this context, so “Runway’s Gen-2” is provided as a more accurate example)
Generative Ai Meaning
AI Gets Creative: Introducing Generative AI
Generative AI, or GenAI for short, is a branch of artificial intelligence with a superpower – it can create entirely new things! From crafting captivating stories to composing music, this AI can generate text, images, videos, and even audio based on what you tell it.
So how does this magic work? Generative AI devours massive amounts of existing content, like text or images. By analyzing these mountains of data, it learns the patterns and structures that make up different types of content. Then, armed with this knowledge, it can create brand new content that feels similar to what it learned from.
Imagine you’re writing a novel. Generative AI can help you craft entire articles or stories based on a simple starting point like a topic or a character description. Feeling artistically blocked? This AI can create realistic images based on your written descriptions, bringing your ideas to life.
The applications of Generative AI extend far beyond creative pursuits. It’s used in fields like entertainment, marketing, and software development to boost creativity and streamline processes. This technology is still evolving, but its potential to unlock new possibilities is undeniable.
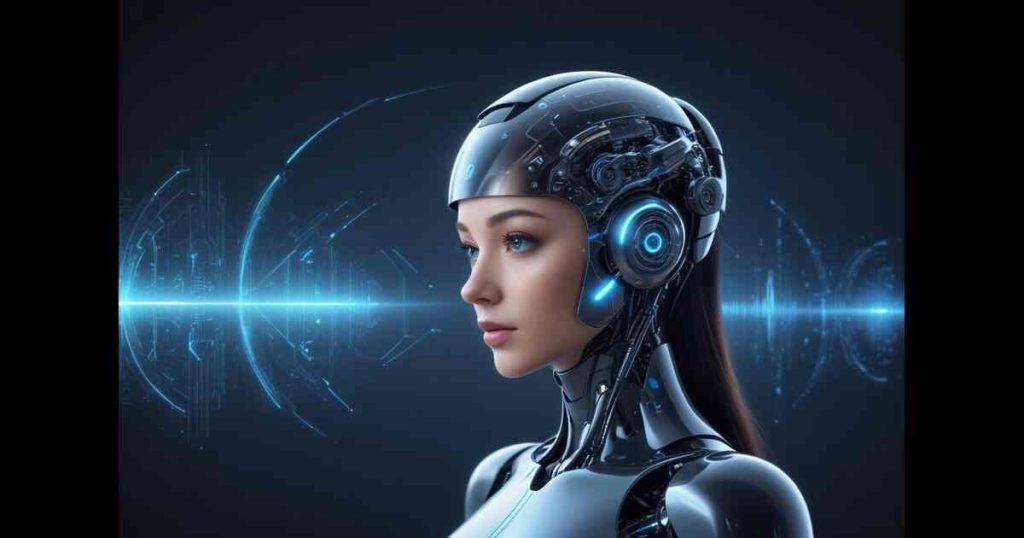
How does generative AI work?
enerative AI operates by leveraging machine learning models, particularly deep learning, to create new content. Here’s a simplified overview of how it works:
- Data Collection: The AI is trained on a vast dataset containing examples of the type of content it aims to generate. For instance, a text-generating AI might be trained on a corpus of books, articles, and other written materials.
- Training the Model: During training, the AI learns patterns, structures, and features from the data. This involves adjusting the model’s parameters to reduce errors in predicting the next piece of data in a sequence.
- Generating Content: Once trained, the AI generates new content by predicting and creating sequences based on the learned patterns. For example, a text generator might predict the next word in a sentence, while an image generator might predict the next pixel or section of an image.
- Fine-Tuning: The model can be fine-tuned on specific tasks or datasets to enhance its performance in particular areas, making the AI more versatile and capable of generating higher-quality content.
- User Input: Generative AI often requires a prompt or input from the user to initiate the generation process. The AI uses this input to guide its content creation.
For example, text generation models like GPT-4 utilize a transformer architecture to understand context and produce coherent and contextually relevant text. Similarly, image generators like DALL-E combine convolutional neural networks (CNNs) and transformers to create detailed and realistic images.
Generative AI refers to a category of artificial intelligence systems designed to generate content that is indistinguishable from content created by humans. This can include text, images, music, and other forms of media. The core technology behind generative AI typically involves neural networks, especially deep learning models such as Generative Adversarial Networks (GANs), Variational Autoencoders (VAEs), and transformers.
Key Concepts in Generative AI:
- Neural Networks: These are computing systems inspired by the biological neural networks of animal brains. They are a key technology in generative AI, particularly deep neural networks.
- Deep Learning: A subset of machine learning involving neural networks with many layers (hence “deep”). Deep learning models have proven particularly effective for generative tasks.
- Generative Adversarial Networks (GANs): These consist of two neural networks, a generator and a discriminator, which are trained together. The generator creates content, and the discriminator evaluates it. The goal is for the generator to create content that the discriminator cannot distinguish from real content.
- Variational Autoencoders (VAEs): These are another type of generative model that learns the underlying distribution of the data and can generate new data points that are similar to the training data.
- Transformers: A type of model that has revolutionized the field of natural language processing (NLP). Transformers can generate coherent and contextually relevant text, and they form the basis for models like GPT-3 and GPT-4.
Applications of Generative AI:
- Text Generation: Creating human-like text for various applications, including chatbots, content creation, and translation services. Examples include OpenAI’s GPT models.
- Image Generation: Creating realistic images from scratch or modifying existing images. GANs are particularly popular in this area, with applications in art, design, and entertainment.
- Music Generation: Composing music that mimics the style of human composers, useful in entertainment and personalized music services.
- Video Game Development: Creating realistic environments, characters, and scenarios in games.
- Drug Discovery: Generating new chemical compounds that could be used in pharmaceuticals.
- Personalization: Crafting personalized content, recommendations, and experiences for users based on their preferences and behavior.
Challenges and Considerations:
- Ethical Concerns: Generative AI can create convincing fake content, leading to issues such as misinformation, deepfakes, and intellectual property concerns.
- Quality Control: Ensuring the generated content is accurate, relevant, and free from biases is a significant challenge.
- Computational Resources: Training and running generative AI models can be resource-intensive, requiring substantial computational power.
Generative AI represents a powerful and rapidly advancing field, with the potential to transform various industries by automating and enhancing creative processes.
Generative AI, short for Generative Artificial Intelligence, refers to a specific type of AI that focuses on creating entirely new content. Unlike traditional AI that analyzes and interprets existing data, generative AI uses its knowledge to produce entirely new things, like:
- Images: Generative AI can create realistic or artistic images from scratch, following your instructions or based on a dataset of existing images.
- Text: This AI can generate different creative text formats, from poems and scripts to realistic news articles or even code!
- Audio & Music: It can compose original music pieces in various styles or even generate realistic human speech.
- Video: Generative AI can create short videos from scratch or manipulate existing ones to create entirely new content.
Here’s a breakdown of how Generative AI works:
- Training: Generative AI models are trained on massive datasets of existing content like images, text, code, or audio. This data gives the AI a deep understanding of patterns, styles, and relationships within that specific type of content.
- Learning: As the AI processes this data, it learns to identify the underlying rules and structures that govern how the content is created.
- Generation: Once trained, the AI can then use its knowledge to generate entirely new content that follows those same rules and structures. It can create something completely original or use your instructions to tailor the generated content to your specific needs.
Some of the benefits of Generative AI include:
- Increased Creativity: This AI can help artists, designers, and content creators explore new ideas and generate fresh content in a fraction of the time.
- Personalization: Generative AI can personalize content experiences, tailoring music recommendations, news articles, or even product designs to individual preferences.
- Efficiency: It can automate content creation tasks, freeing up human resources for more complex work.
However, there are also some potential challenges associated with Generative AI:
- Bias: If the training data used to create the AI model is biased, the generated content can also be biased.
- Misinformation: The ability to create realistic-looking fake content raises concerns about the spread of misinformation.
- Job displacement: As AI automates content creation tasks, it could potentially lead to job losses in certain sectors.
Overall, Generative AI is a rapidly evolving field with the potential to revolutionize the way we create and consume content. As with any new technology, it’s important to be aware of both the benefits and challenges associated with it.
Pingback: Generative AI: A New Era of Content Creation – "Xelent Solutions: Empowering Your Digital Journey with Web and App Development"